Maximizing Efficiency: Machine Learning for Predictive Maintenance in Industrial IoT Systems
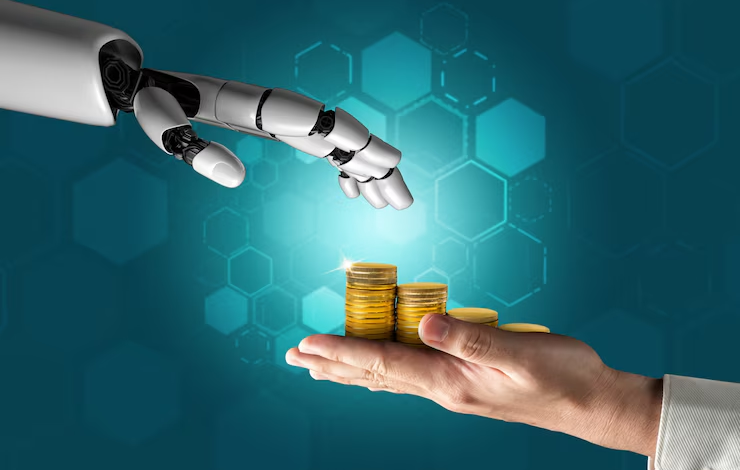
In industrial operations, ensuring the uninterrupted functionality of machinery and equipment is paramount for maintaining productivity, reducing downtime, and optimizing operational costs. Traditional maintenance practices often rely on scheduled inspections or reactive repairs, which can lead to inefficiencies and unexpected breakdowns. However, with the advent of Industrial Internet of Things (IIoT) systems and machine learning technologies, predictive maintenance has emerged as a powerful strategy for proactively identifying equipment failures and optimizing maintenance schedules. In this article, we delve into the role of machine learning in predictive maintenance for IIoT systems and its implications for industrial efficiency and reliability.
Contents
The Evolution of Predictive Maintenance:
Predictive maintenance involves using data analytics and machine learning algorithms to predict when equipment failures are likely to occur, enabling proactive maintenance actions to be taken before failures occur. Traditionally, predictive maintenance relied on manual inspections, sensor readings, and historical data analysis to identify signs of equipment degradation or malfunction. However, with the proliferation of IoT devices and sensors and advancements in machine learning algorithms, predictive maintenance has become more sophisticated and accurate. Mpc wallet ensures secure transactions within the IIoT ecosystem, facilitating seamless financial interactions for industrial operations.
Harnessing Data Analytics and Machine Learning:
Machine learning plays a central role in predictive maintenance by analyzing vast amounts of sensor data, equipment telemetry, and operational metrics to identify patterns and anomalies indicative of impending failures. Supervised learning algorithms, such as classification and regression models, can be trained on labelled data to predict equipment failures based on historical patterns and known failure modes. Unsupervised learning algorithms, such as clustering and anomaly detection, can also be used to identify abnormal behaviour and deviations from normal operating conditions. By leveraging data analytics and machine learning, industrial organizations can gain valuable insights into equipment health and performance, enabling proactive maintenance interventions to prevent costly downtime and repairs.
Optimizing Maintenance Strategies:
Predictive maintenance enables industrial organizations to optimize their strategies by prioritizing maintenance activities based on equipment health and criticality. By accurately predicting when equipment failures are likely to occur, maintenance teams can schedule preventive maintenance tasks during planned downtime, minimizing disruption to production schedules and avoiding unplanned shutdowns. Additionally, predictive maintenance allows maintenance resources to be allocated more efficiently, ensuring that maintenance activities are focused on the most critical assets and components. This optimization of maintenance strategies leads to improved asset reliability, reduced maintenance costs, and increased operational efficiency.
Reducing Downtime and Operational Costs:
One of the primary benefits of predictive maintenance is its ability to reduce downtime and operational costs by proactively addressing equipment failures before they occur. By identifying potential issues early on, maintenance teams can take corrective action to prevent equipment breakdowns and production stoppages. This not only avoids the costs associated with unplanned downtime, such as lost production, idle labour, and emergency repairs but also extends the lifespan of equipment and reduces the need for costly replacements. Furthermore, predictive maintenance enables organizations to transition from reactive to proactive maintenance, leading to overall cost savings and improved bottom-line performance.
Embracing a Culture of Continuous Improvement:
In conclusion, machine learning for predictive maintenance in IIoT systems empowers industrial organizations to embrace a culture of continuous improvement and operational excellence. Organizations can proactively identify and address equipment failures, optimize maintenance strategies, and minimize downtime and operational costs by leveraging data analytics and machine learning algorithms. With innovative solutions like mpc wallet supporting secure transactions within the IIoT ecosystem, industrial organizations can unlock the full potential of predictive maintenance to drive efficiency, reliability, and competitiveness in today’s fast-paced industrial landscape. As machine learning continues to evolve, the future of predictive maintenance holds promise for even more significant advancements in industrial efficiency and performance.